SINTRA
An upcoming ITEA project poised to revolutionize the protection of critical infrastructure. As we embark on this journey, SINTRA aims to enhance resilience by creating an open data-streaming AI platform. Through advanced multi-modal sensing and AI analysis, it will offer a comprehensive view of safety and security, enabling stakeholders to proactively detect and respond to complex anomalies. Stay tuned as SINTRA paves the way for a more secure and interconnected future.
Project Introduction
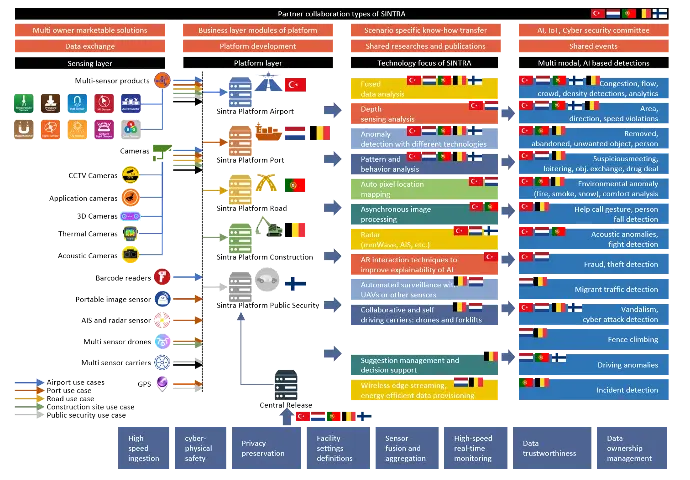
However, the capabilities of these solutions are insufficient to ensure resilience and protection of critical infrastructure. Lack of trustworthy means for public-private cross-coordination, low interoperability and weak compliance with the EU data-privacy legislation are leading to local-only deployment of these systems and, as a result, fragmented situational awareness of security operators. Decisions are currently based on fragmented information within closed systems and siloed organization models. Besides this, the common reliance on analysis of sole video data limits the monitoring to simple incidents (trespassing, panic, fighting), but does not allow detection of complex, high-impact, and context-dependent threats (human/drug trafficking, thievery, attacks on infrastructure).
The SINTRA project aims to overcome these limitations by delivering an open data-streaming AI platform that enables cross-organizational interoperability and ensures trustworthiness in the safety and security monitoring. The platform facilitates cross-coordination between involved stakeholders, aids information sharing, management, and analysis from the public and private security operators, thereby enabling global situational awareness in the infrastructure threats. SINTRA aims at researching and defining the methodology for EU legislation-aware privacy protection and ethical use of data, that serves as a basis for the cross-coordination.
Technology-wise, the project envisions a significant step beyond the state-of-the-art by the synthesis of innovative multi-modal sensing and AI-powered combined data analysis. Incorporation and fusion of acoustic, visual, radar, multispectral, LiDAR, ToF or environmental sensor modalities together with already existing data sources (police data, logistic timetables, social media data) helps to obtain a multi-faceted, comprehensive view on the security/safety of the infrastructure situation. The AI-based analysis of the combined data enables robust detection of hidden, complex, or context-dependent anomalies, as well as their subsequent mapping to threats and timely cross-coordinated response, contingency or mitigation.
The SINTRA consortium is composed of partners from six countries (The Netherlands, Turkey, Belgium, Finland, Portugal, and Germany) that cover the full market value chain of research centers, sensor/data providers, platform, and service providers, where each country use-case is supported by one or more end-users. The consortium carefully balances the scale and impact of large industrial partners () providing the platform and service integrations with the in-depth expertise of academic institutes () and the innovative power of selected SMEs (). The benefits of the SINTRA platform will be demonstrated on six critical infrastructure types of end-users: logistic hubs (Port of Moerdijk), airports (), harbors, construction sites, shopping centers, and road networks. The project will actively engage with citizens, authorities, and external stakeholders to stimulate acceptance, validate scalability, and maximize the impact.
The expected project business impact is threefold. First, the current analysis-based security industry is technologically stagnating due to the constantly rising legislation barriers on data collection and usage for machine learning. Establishment of the methodology for privacy-preserving AI-based security systems will enable large-scale business growth in this domain. Second, the plug-and-play SINTRA platform will help to reduce the partner maintenance and technology upgrade costs by up to 120m euro a year. Finally, and most importantly, the project results allow partners to enter the opening market of full-fledged security and monitoring solutions, with additional revenues estimated to 400m euro a year.
Process
Born of Idea
At the PO Days event organized by ITEA in Helsinki, two projects were merged, journey started.
Po Submitted
Po Approval
FPP Submitted
FPP Approved
First Country Approval
Netherlands consortium got approval from their national funding agency
First CR Approval
Other Countries Approval
Except Finland, all countries got approval but Finnish partners started to project as self-funding organizations
Project Start
Kickoff Meeting
PCA Signatures
WP1 Started
Other work packages started
Focused Challenges
SINTRA open data-streaming AI platform enables
interoperability and trustworthiness in the communication and AI data
processing and facilitates cross-coordination between tenants, sensors, and
systems. In this project, we collect the real-world view on the current
constraints, limitations, possibilities and needs for cross-coordination from
our stakeholders and build trustworthy protocols and means for data exchange
between security organisations.
The SINTRA platform enables high situational awareness for the security personnel by integrated visualisation layer that renders all important and critical information on one display. Thus, operators will not have to experience switching between screens or systems and glaze at hundreds of monitors at the same time. In addition, the anomaly detection engine proposed in the SINTRA project scenarios will reduce the volume of information that the security officers need to examine.
Research conducted during the project preparation
period has shown that the literature on anomaly detection remains limited,
especially in the project-related industries and specific scenarios. Within the
scenarios, sensor fusion and multimodality solutions will be developed to form
an accurate context understanding by enabling dynamic and autonomous use of the
available or built-in sensors and data streams. During the project, several
AI-based detector algorithms are planned to be developed for the following
safety threats, crime activities and anomalies: accident, collision, anomalies
in human/vehicle/vessel behaviours, subversive crime, drugs dealing and
trafficking, people smuggling, burglary, cargo thievery, robbery, fraudulent
waste, suspicious object exchange, infrastructure attacks (digging, fence
cutting) congregation, dwell time anomalies, trespassing, suspicious meetings,
fights, coughing and drowning detection.
Anomaly definitions can differ depending on the
actor’s location and activity history, season, day of week, density of people,
etc. Therefore, SINTRA tackles anomaly/threat detection in a context-based
manner, where the context is obtained by analysis of data from integrated
sensors or external data sources, such as AIS, GIS, weather services, calendar,
and other operational systems.
At the initial project phases, we perform the GDPR
legislation analysis and map the legal requirements on our data types and processing
chain. Solutions such as edge analytics, data anonymization, data filtering,
homomorphic encryption and federated learning are already foreseen within the
scope of the project scenarios.
Explainability, privacy protection, robustness,
fairness/no-bias, privacy protection and transparency are the trustworthiness
dimensions of any AI system. They are vital to enable cross-coordination of
stakeholders. Therefore, SINTRA focuses on these characteristics during
algorithmic development by iteratively assessing the dimensions during the
project and involving the researchers, regulators, end-users, and stakeholders for iterative algorithmic improvement
on compliance.
The project envisions research on automated and
self-coordinating sensor carriers (UAV, rovers). This concept enables the
possibility for systematic coverage of ‘dead zones’ and obtaining
high-resolution real-time data from the areas featuring suspicious activities.
The sensor carriers will be integrated into the architecture and should require
little efforts on manual guidance, flight, and camera control.
The open platform architecture designed for interoperability allows easy and dynamic integration of new sensors, analysis tools and communication protocols into an existing system. This largely reduces costs for future maintenance and upgrades.
With respect to operational costs, the following proposed solutions lead to the efficient use of ICT infrastructure and personnel efforts.
● Expected impact of SINTRA is to decrease crime protection costs for involved stakeholders by better cooperation, data exchange and response protocols.
● Deployment of dynamic sensor carriers not only provides better area monitoring coverage, but also reduces the installation and maintenance costs compared to statically located sensors.
● The proposed CCTV smartening solution will generate cost efficiency: asynchronous image processing methodology driven by sensor-based anomaly detection.
● In several scenarios, partners will aim to reduce operational costs with the predictive maintenance and accident prediction solutions.
● In
order to reduce costs, edge processing methods will be applied, combined with
reliable energy-efficient data provision of wireless sensory and edge systems.
Processing power requirements, insufficient
resource capacity needs are the problems to be experienced both in the platform
and in the subsystems that will integrate with the platform. The solution of
the problem on the platform side is explained in another Problem Definition
section. On the subsystem-level, available processing capabilities (subsystem
cores) will be utilised for effective data pre-processing and reduction
techniques (edge pre-processing) to lower processing efforts on subsequent
platforms and cores in the data stream.
Sensors located on the Object Under Supervision
(SUP) and their wireless transmission channels are a first obvious
vulnerability to adversarial attacks of the whole system. To counter these,
multimodal sensor data with partially redundant observation capabilities are a
first counter measurement by concept. Furthermore, resilience concepts will be
integrated by redundant data transmission channels, which can be
selected/deselected at runtime, based on real-time feedback on coverage,
disturbance, interference, and breakdown appearances. The channel analyses and
feedback can be realised as part of the system diagnose feature.
Due to the multimodal sensing strategy and the
partially redundant and partially complementary observation data from different
sensors and sensor modalities, biases, quantization effects and precision
errors are conceptually tackled by sensor fusion techniques. They should
furthermore address overfitting due to decreased signal variability
(uncertainty) and an increased diversity (complementary effects) of different
sensor modalities and especially from resulting combinations.
Combined analysis of multi-modal sensor data always
exposes challenges on data Representation, Translation, Alignment, Fusion, and
Co-learning. For the sensor/data set foreseen in SINTRA, a very limited
literature on the data transformation is available. It is planned to develop a
“Data transformation for standardisation” module within the Business Layer of
the platform. Existing standards for sensor data that could be applied to
transfer information into common representations will be adopted. In addition,
novel methods for previously unexplored transformation/fusion of data
modalities will be researched and developed.
The automated activity recognition (machine learning)
poses a possible vulnerability of the system, due to false positives and false
negatives. These typically result from a limited amount of training data, high
variability in processes to be recognized, as well as missing information.
These problems are conceptually tackled with the planned multimodal sensing
strategy, allowing for a higher diversity in observation information of the
same process, as well as reduced signal variability (uncertainty) due to
complementary observations from multiple sensor modalities.
The envisioned functions of the SINTRA platform are
presented in section 2.3.3 in detail. To summarise, necessary isolation studies
will be carried out on all platform layers to ensure tenants' privacy. In addition,
at the Business Layer, the functional modules required to meet the needs of
data ownership management, data source management, and trustworthiness are
placed in the architecture. Also, to solve the problems observed in every big
data platform, such as velocity, variety, and volume, a structure was designed
in which only the analyses obtained from sensors are transferred into the
system instead of entire sensor data. For example, sending camera images to the
platform as it is, will push the system's capacity limits. Moreover, dynamic
capacity distribution between tenant operations and standardisation modules to
ease sensor fusion activities are defined in the architecture.
In safety and security operations, estimations related
to operation inputs are necessary for adequate workforce planning. For example,
for the airport, estimation of the number of passengers arriving at the
terminal on the day of operation, the number of aircraft to land, the amount of
luggage of passengers, time-based arrival patterns of passengers determine how
many monitoring officers will be required in the relevant operation period.
Relevant estimations can only be achieved with data from other systems in the
operations ecosystem. Integrations with relevant operational systems have been
planned within the scope of scenarios to increase predictability. In addition,
in some scenarios, what-if and trend analysis will be developed to contribute
strategic planning. From the safety perspective, analyses such as predictive
maintenance and incident prediction are defined in some sample scenarios within
the scope of SINTRA.
Involved Countries and Partners
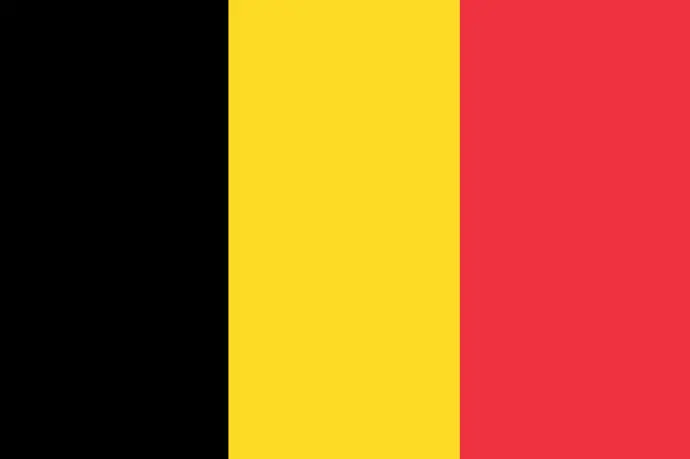
Belgium
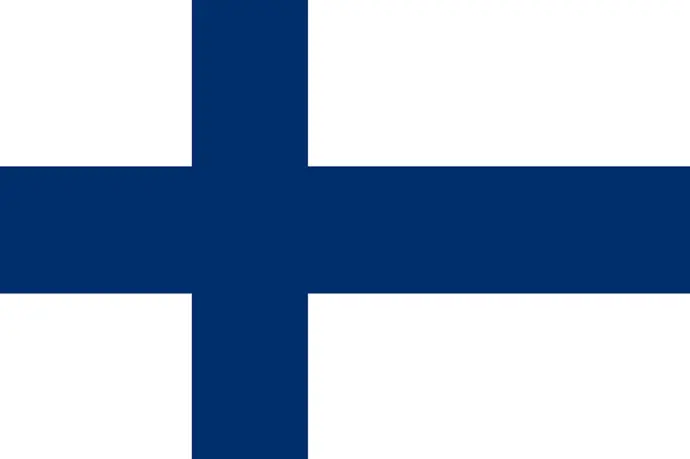
Finland
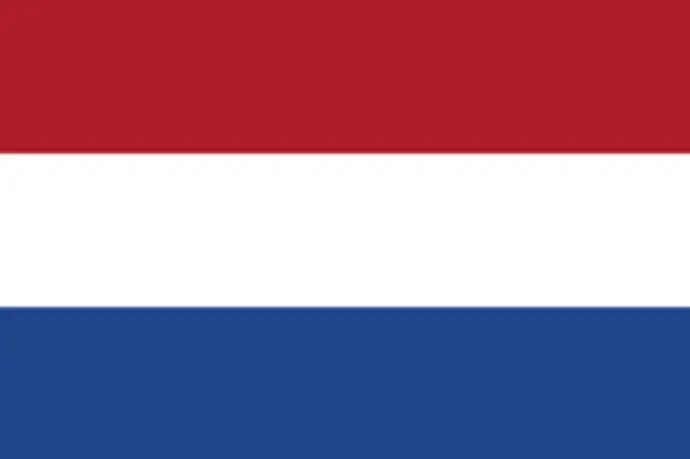
Netherlands

Turkey
Project Plan
Workpackages